AI's Potential Ouroboros Problem
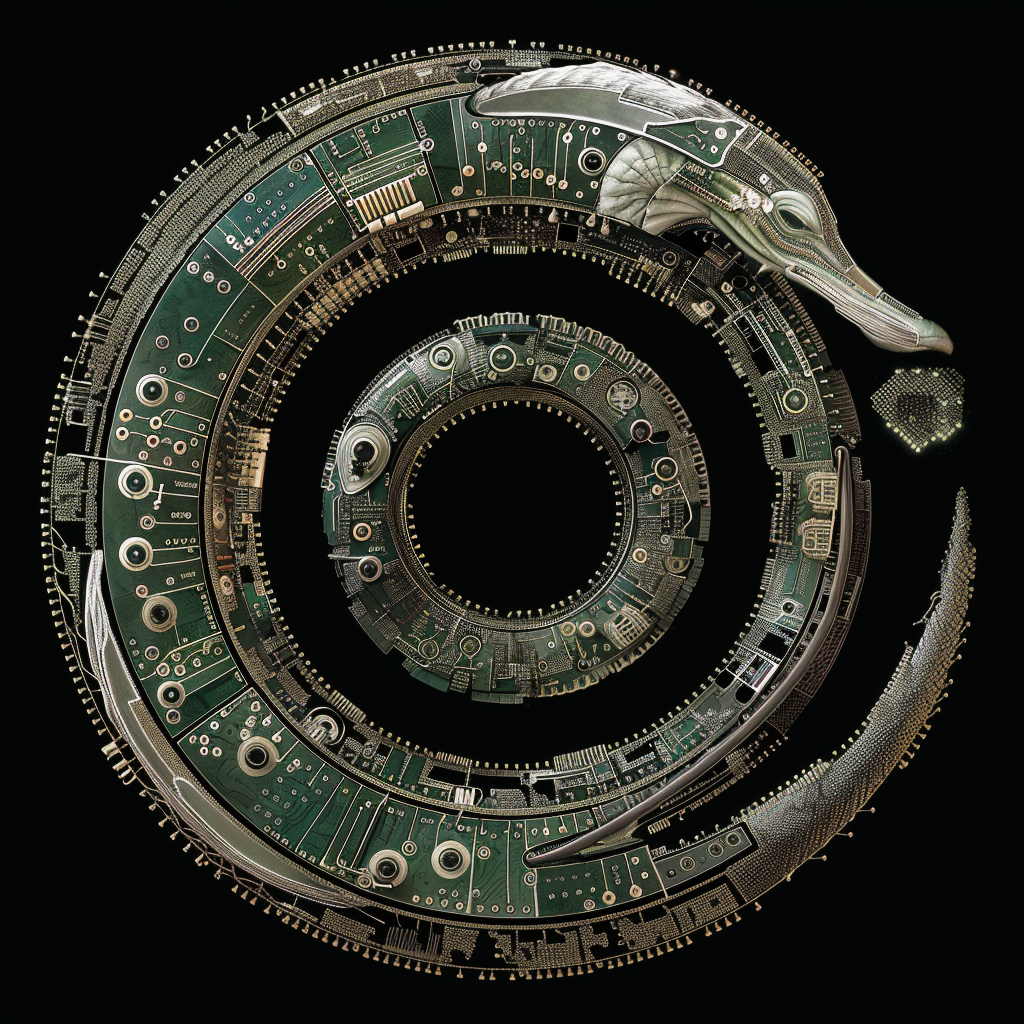
Admittedly, this is a bit above my pay grade, but in thinking about and writing about AI over the past many months, my mind keeps going back to a place where I'm mildly disturbed by the concept of "synthetic data" (see: my notes below). Specifically, how because we're running out of "natural" data to train AI models, many are now being trained on data created by other models.
Conceptually, I understand why it should work. And see how, in many ways, it might yield cleaner inputs. But it also just feels like one of those things that is unnatural. Which I realize is silly to say in relation to AI. Still, here we are.
From a report today by Michael Peel in The Financial Times:
Research published in Nature on Wednesday suggests the use of such data could lead to the rapid degradation of AI models. One trial using synthetic input text about medieval architecture descended into a discussion of jackrabbits after fewer than 10 generations of output.
Yes, we've moved on from discussions of llamas to jackrabbits today. Yes, this is hilarious. But also mildly concerning because again, this is the high-level method a lot of our current models are going to need to train on to keep growing, if they're not already. And yes, this study was done for research purposes not using the actual data inputs the model makers are using, but it points to something that seems pretty fundamental with enough synthetic data going in...
“Synthetic data is amazing if we manage to make it work,” said Ilia Shumailov, lead author of the research. “But what we are saying is that our current synthetic data is probably erroneous in some ways. The most surprising thing is how quickly this stuff happens.”
The paper explores the tendency of AI models to collapse over time because of the inevitable accumulation and amplification of mistakes from successive generations of training.
The speed of the deterioration is related to the severity of shortcomings in the design of the model, the learning process and the quality of data used.
The early stages of collapse typically involve a “loss of variance”, which means majority subpopulations in the data become progressively over-represented at the expense of minority groups. In late-stage collapse, all parts of the data may descend into gibberish.
I'm reminded of The Architect speech in The Matrix: Reloaded where he talks about the creation of the "perfect" system only for it to collapse as the system rejected the unnatural perfection. But it's not just gibberish we need to worry about, it's arguably madness.
The researchers found the problems were often exacerbated by the use of synthetic data trained on information produced by previous generations. Almost all of the recursively trained language models they examined began to produce repeating phrases.
In the jackrabbit case, the first input text examined English church tower building during the 14th and 15th centuries. In generation one of training, the output offered information about basilicas in Rome and Buenos Aires. Generation five digressed into linguistic translation, while generation nine listed lagomorphs with varying tail colours.
Here, I'm reminded of Leonardo DiCaprio playing Howard Hughes in The Aviator, just standing there repeating "the way of the future" over and over and over again.
Another example is how an AI model trained on its own output mangles a data set of dog breed images, according to a companion piece in Nature by Emily Wenger of Duke University in the US.
Initially, common types such as golden retrievers would dominate while less common breeds such as Dalmatians disappeared. Finally, the images of golden retrievers themselves would become an anatomic mess, with body parts in the wrong place.
Now I'm reminded of some sort of badly failed cloning process we see so often portrayed as something slightly off devolving into well, a mess – Alien: Resurrection? The Rise of Skywalker? Alright, I'm stretching this gimmick too far. But here may be why this all actually matters:
“One key implication of model collapse is that there is a first-mover advantage in building generative AI models,” said Wenger. “The companies that sourced training data from the pre-AI internet might have models that better represent the real world.”
In other words, there's a risk that the players who are already established as the model makers are the only ones we're ever going to get. Because their models have subsequently flooded the internet with junk data breeding like jackrabbits.
Again, I understand there's quite a bit of caution being used with this "synthetic data" for obvious reasons. At the same time, my mind wanders to the paleontologists seeing the lab at Jurassic Park for the first time and are told by Dr. Henry Wu that there's no need to fear the dinosaurs reproducing in the wild because all of the dinosaurs have been created as female. To quote Dr. Ian Malcolm perhaps in actual context for the first time on the internet, "Life, uh, finds a way."
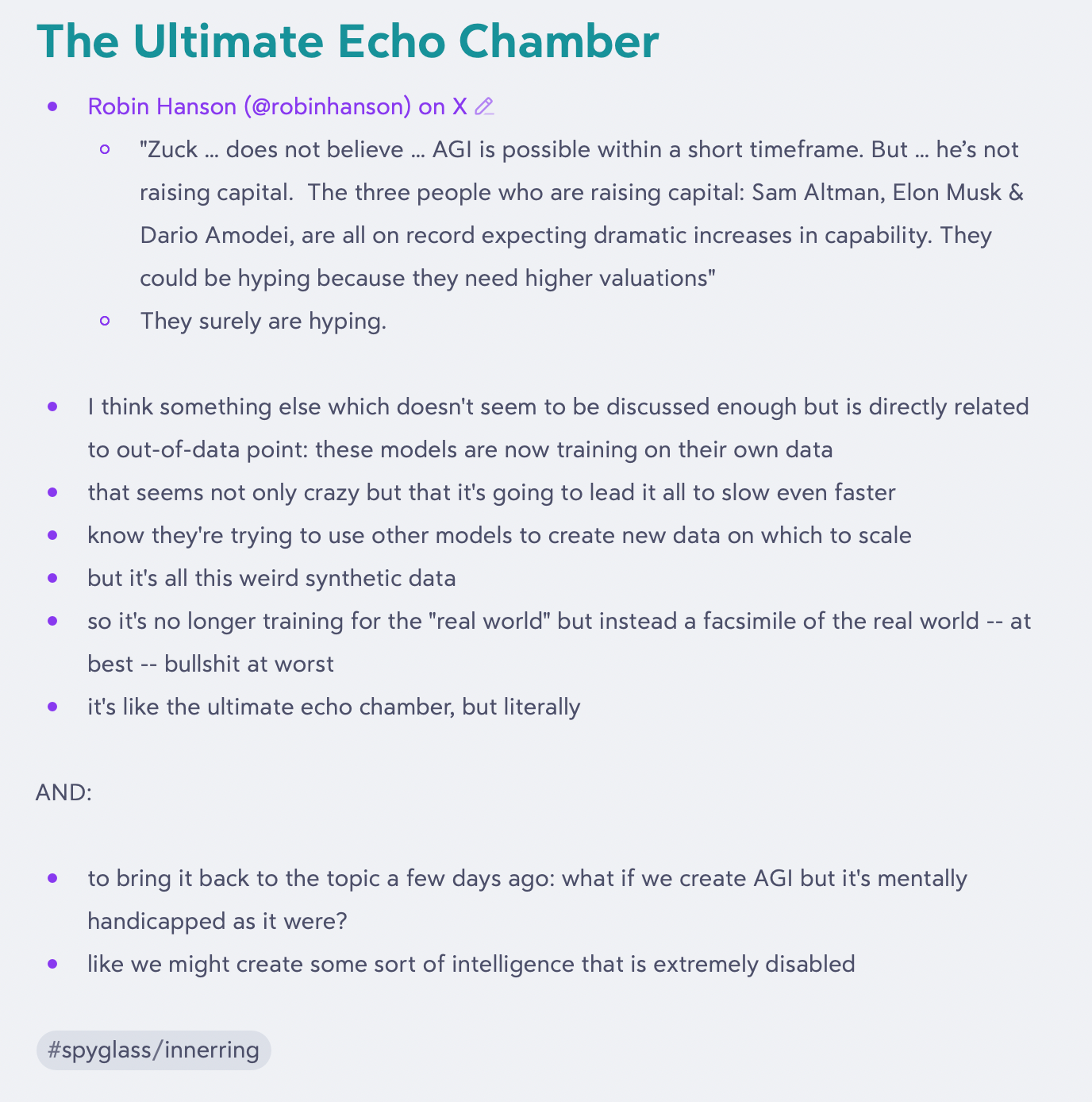
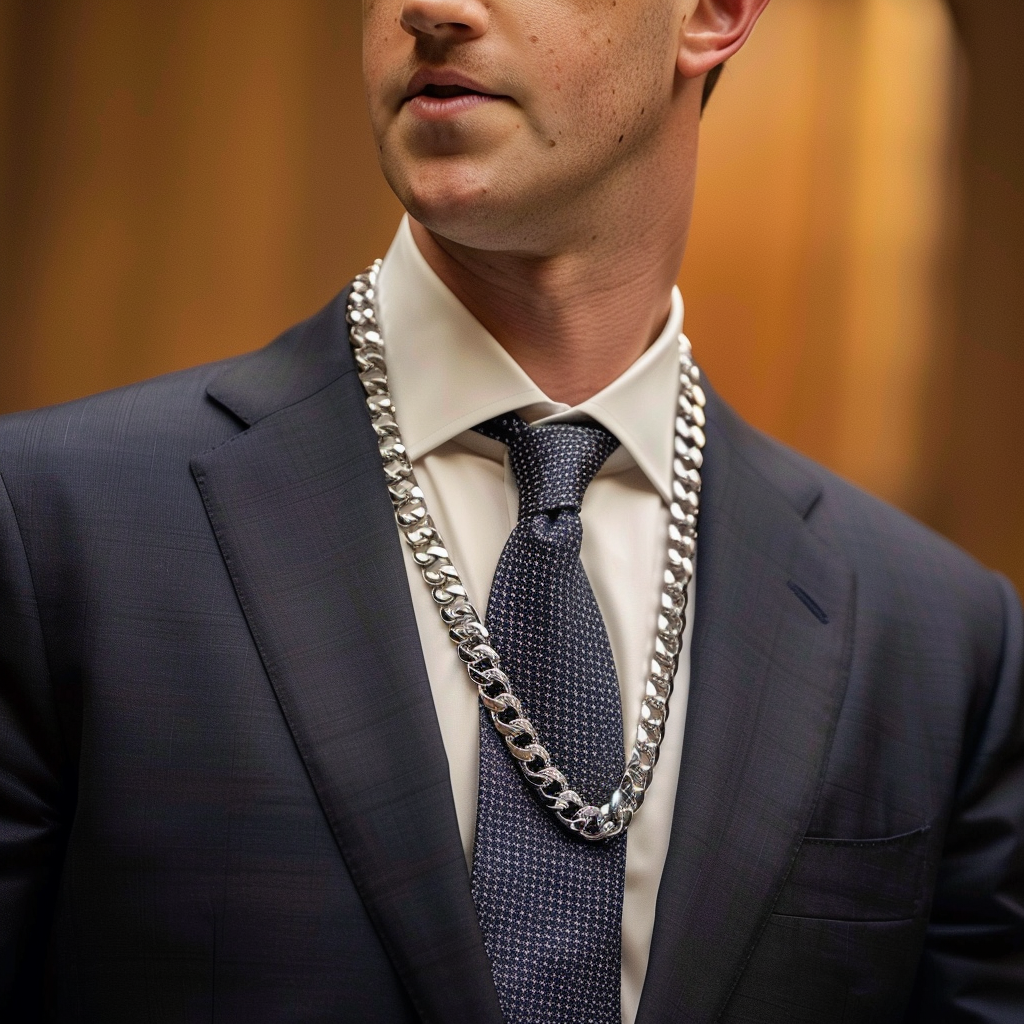
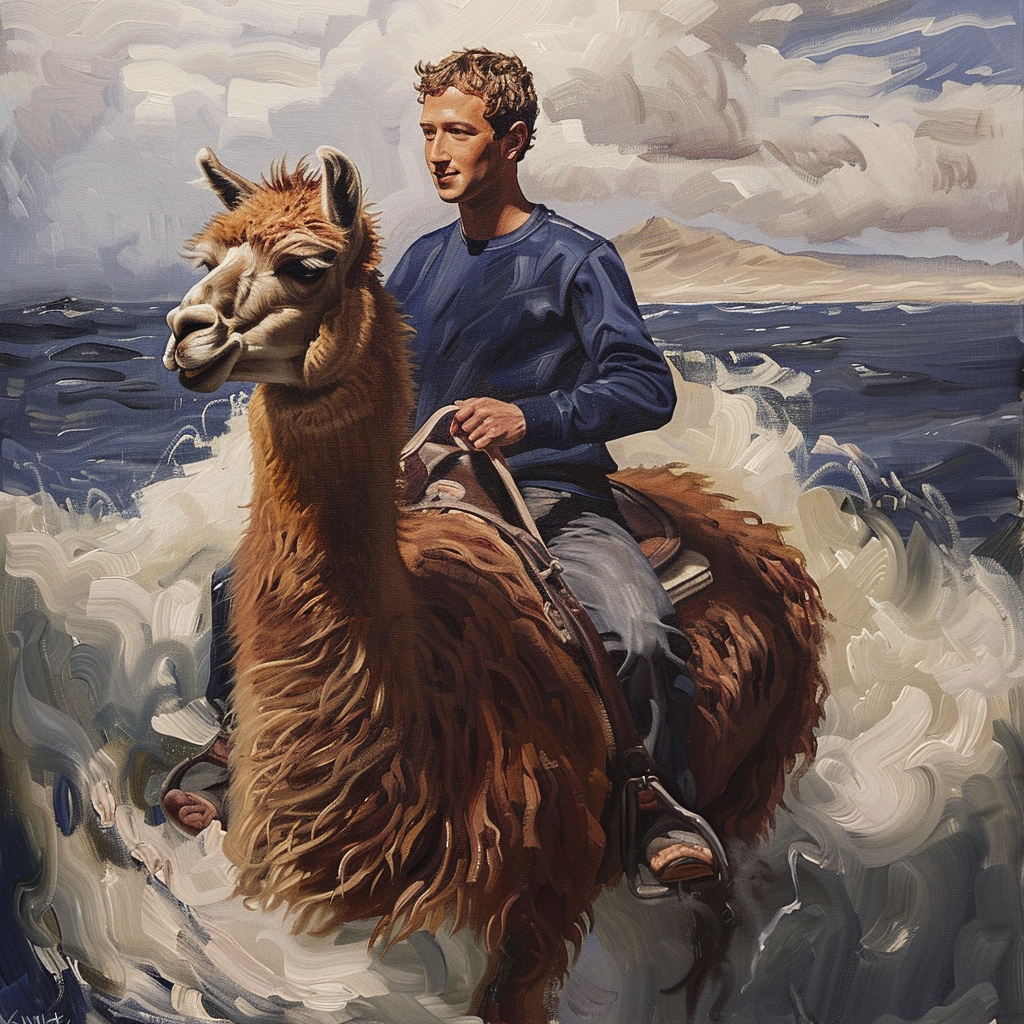